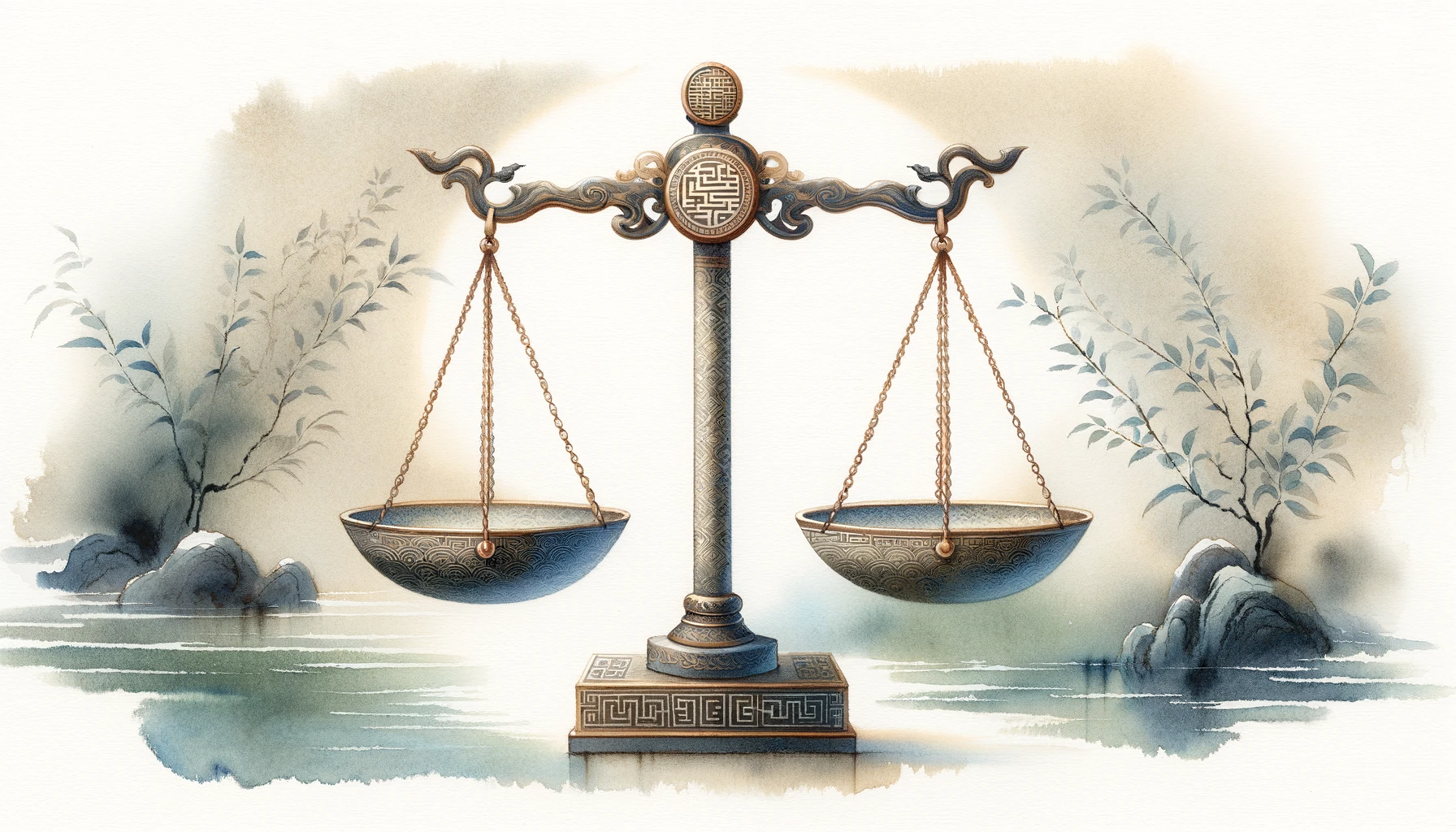
How to Measure LLM App Engagement Metrics
In the dynamic field of AI product development, the key to success lies in accurately gauging and enhancing user engagement. While developers may have assumptions about user preferences, actual user behavior and preferences can differ significantly. Employing a robust framework for tracking and analyzing user engagement metrics is essential to refine and elevate the product experience.
The Critical Role of Utility in LLM Features
The investment in Language Learning Models (LLMs) necessitates a rigorous assessment of their utility. This evaluation is two-fold: through an Overall Evaluation Criteria (OEC) for a holistic assessment, and specific engagement metrics for detailed analysis. This dual approach ensures that each feature not only aligns with the product’s overall objectives but also resonates with the end-user.
Understanding of Overall Evaluation Criterion (OEC)
OEC is a nuanced, composite quantitative measure that encapsulates the experiment’s goals. It often combines multiple Key Performance Indicators (KPIs) to form a unified metric. This comprehensive measure is preferred when singular metrics fall short in evaluating the experiment’s outcome. A well-constructed OEC should encompass factors predicting long-term success, like customer lifetime value, rather than just short-term gains. It aligns the entire organization towards a unified, long-term objective.
User Engagement & Utility Metrics
Comprehensive Metrics
–Visited: This metric goes beyond mere counts, analyzing the frequency and duration of app or feature visits, offering insights into initial attraction and ongoing engagement.
–Submitted: A deeper look into the types of prompts submitted by users can reveal their needs and expectations from the LLM.
–Responded: Beyond counting responses, assessing the relevance and helpfulness of these responses is critical in determining the LLM’s effectiveness.
–Viewed: This involves understanding not just if a response is viewed, but how users interact with it – do they share it, reference it, or ignore it after viewing?
–Edited: Tracking edits provides insights into user satisfaction and the adaptability of the LLM to user preferences.
–Rated: User ratings, coupled with qualitative feedback, offer a rich understanding of user satisfaction.
–Saved: The context in which responses are saved and retrieved later can indicate the long-term value users find in the LLM’s output.
Enhancing User Experience through Targeted Engagement Analysis
Analyzing which app features captivate users and which fall short is key to developing a successful product. This involves not just identifying popular features but also understanding why they succeed. Similarly, understanding the shortcomings of less popular features can lead to significant improvements.
Tailoring Engagement Strategies to Business Models
The definition of user engagement varies across different business models. For an e-commerce platform, metrics like cart additions and page views per session are critical. For a content-driven app, the focus might be on content interaction and sharing. Aligning the engagement strategy with these specific business goals is paramount.
Merging Traditional Metrics with Product Experience Insights
Traditional web metrics such as bounce rates and session duration provide a foundational understanding of user behavior. However, integrating these with product experience (PX) insights, such as user journey mapping and sentiment analysis, offers a more comprehensive picture of user engagement.
Expanding on Additional User Engagement and Utility Metrics
Opportunities and Visibility
- Opportunities to Suggest Content: This metric tracks the total instances where the LLM is activated, regardless of whether users are aware of it. It’s a measure of how often the LLM is utilized, providing insights into its integration in the user experience.
- Prompts to LLM: Captures the total number and frequency of prompts made by users. This metric is vital to understanding user reliance on and engagement with the LLM.
- Responses from LLM: Monitors the total number and frequency of responses generated by the LLM. It’s an indicator of the LLM’s responsiveness and its capacity to keep users engaged.
- Responses Seen by Users: This metric measures the total number and frequency of responses that are actually displayed to users, considering factors like moderation and relevance. It helps evaluate the effectiveness of the LLM in delivering pertinent information to users.
User Interaction
The suite of “User Interaction” metrics plays a pivotal role in deciphering the effectiveness and alignment of these advanced systems with user needs. Key among these metrics are the User Acceptance Rate, which illuminates how frequently users accept the LLM’s responses, providing an indication of how well these responses meet user expectations in various contexts. Complementing this, Content Retention metrics offer a window into the lasting impact of the LLM, measuring how much of its output is retained by users over time. Together, these metrics provide invaluable insights into user satisfaction and the enduring value derived from interacting with the LLM, making them essential for refining and enhancing the user experience.
- User Acceptance Rate: Tracks the frequency of user acceptance, which varies by context, such as text inclusion or positive feedback in conversational scenarios. This metric is crucial for understanding how well the LLM’s responses align with user expectations.
- Content Retention: Measures the number and rate of LLM-generated content retained by users over a specified period. It provides insights into the long-term value users find in the LLM’s output.
Quality of Interaction
- Prompt and Response Lengths: Analyzes the average lengths of prompts and responses, offering insights into how in-depth the interactions between users and the LLM are.
- Interaction Timing: This metric captures the average time between prompts and responses and the time users spend on each, providing a measure of engagement and LLM efficiency.
- Edit Distance Metrics: Monitors the average edit distance between user prompts and between LLM responses and retained content. This metric is indicative of the level of prompt refinement and content customization.
Feedback and Retention
“Feedback and Retention” metrics emerge as a fundamental aspect for understanding and enhancing user experience. These metrics encompass User Feedback, which involves tallying responses with positive or negative reactions, providing direct insights into user satisfaction and identifying any potential biases. Conversation Metrics further deepen this understanding by analyzing the length, duration, and frequency of interactions with the LLM, offering a panoramic view of user engagement over time. Complementing these, User Retention metrics, which track daily active users and the retention rates of new users, along with their engagement in initial sessions, are critical for assessing user loyalty and the product’s initial attractiveness. Together, these metrics form a robust framework for evaluating the long-term engagement and satisfaction of users with LLM products, guiding strategies for continuous improvement and user-centric development.
- User Feedback: Counts the number of responses with Thumbs Up/Down feedback, highlighting user satisfaction and potential bias due to low response rates.
- Conversation Metrics: Includes average length and duration of LLM conversations, the number of conversations, and active days using LLM features, giving a comprehensive view of user engagement over time.
- User Retention: Measures daily active users, the retention rate of new users, and first-session feature usage, crucial for understanding user loyalty and the initial appeal of the product.
Creator Productivity Metrics
“Creator Productivity Metrics” play a pivotal role in quantifying the impact of LLMs on content creation processes. These metrics focus on evaluating the efficiency and quality enhancements in content creation facilitated by LLMs. They encompass a broad range of factors, including the speed of content generation, the number of users and sessions engaged in creating content per document, and the extent of document edits made with LLM assistance. Additionally, these metrics delve into the details like the total characters retained by users, the length of their interactions with the LLM, and the balance between user and LLM edits. They also consider the incorporation of rich content elements such as images and charts. Another crucial aspect is the Editing Effort metric, which measures the average time users spend in editing mode, offering insights into the efficiency and user-friendliness of the LLM-supported editing process. Collectively, these metrics provide a comprehensive understanding of how LLMs enhance creator productivity, guiding further optimizations to maximize the utility and effectiveness of these AI-driven tools.
- Content Creation Efficiency: Evaluates the improvement in content creation speed and quality with LLM assistance, reflecting the LLM’s effectiveness in enhancing user productivity.
- Content Reach and Quality: Assesses the number of users and sessions involved in creating content per document, the number of documents edited with LLM assistance, and the total characters retained per user. It also measures the number of total and user edits, and the inclusion of rich content elements like images and charts.
- Number of users and sessions creating content per document.
- Number of documents edited with LLM assistance.
- Total characters retained per user and length of LLM interactions.
- Number of total and user edits, and use of rich content elements like images and charts.
- Editing Effort: Tracks the average time spent by users in editing mode, providing insights into the efficiency of the content creation process.
Consumer Productivity Metrics
“Consumer Productivity Metrics” stand as a crucial indicator of how these technologies influence end-user experiences, particularly in content consumption. This set of metrics assesses various dimensions of how users interact with content that has been enhanced or generated by LLMs. Firstly, Content Consumption Efficiency provides insights into how the use of LLMs affects the speed and quality with which users consume content, reflecting on the LLM’s role in enhancing user comprehension and engagement. The scope extends to Content Reach and Quality, which tracks the number of users engaging with the LLM-enhanced content, the frequency of their interactions, and activities such as sharing and commenting, thus offering a window into the broader impact and appeal of such content. Finally, Consumption Effort delves into the time users invest in consuming this content, a measure that helps gauge overall user engagement and the intuitiveness of content consumption in the context of LLM enhancements. These metrics collectively provide a nuanced view of the user-side efficiency and effectiveness of LLM applications in content-oriented settings.
- Content Consumption Efficiency: Evaluates the speed and quality of content consumption for documents edited with LLM assistance, reflecting the LLM’s impact on user reading and understanding.
- Content Reach and Quality: This metric covers the number of users and sessions consuming content per document, the number of documents read that were edited with LLM, and consumption actions like sharing and commenting per AI-edited document.
- Consumption Effort: Measures the average time spent in consumption mode per document per user, offering insights into the user engagement and ease of content consumption.
User Engagement and Satisfaction: A Strategic Overview
In our endeavor to optimize Language Learning Models (LLMs), a strategic focus is placed on monitoring user engagement. This encompasses assessing the frequency and quality of interactions with LLM features, as well as predicting the likelihood of their future usage. Such an approach is crucial in aligning LLM functionalities with user expectations and enhancing overall satisfaction.
Decoding the Prompt and Response Funnel
Other possible metrics to look at is a detailed analysis of the stages of user interaction with LLMs, ranging from the initial prompt submission to the eventual acceptance or rejection of responses. This analysis is pivotal in understanding not only the utility of LLM responses in specific tasks but also in tailoring them to meet user needs more effectively.
Assessing the Quality of Engagement
The quality of user engagement stands as a critical metric in our evaluation process. By examining aspects like the length of prompts and responses, the edit distances in user interactions, and user feedback ratings, we gain insights into the efficacy of the LLM’s interactions. This holistic view aids in refining response quality as well as created a singular aggregated metric.
Tracking Retention Metrics for Sustained Engagement
An essential aspect of LLM app measurement is a focus on retention, particularly to identify potential declines in user engagement post-initial usage. Retention metrics should be specifically tailored to the nuances of LLM features, enabling you to adapt strategies for sustained user interest and engagement over time.
Enhancing Productivity in Collaborative Environments
In collaborative scenarios involving AI-generated content, you should place a significant emphasis on evaluating productivity enhancements. By analyzing metrics on both the creation and consumption ends, you should aim to quantify the added value of AI content in collaborative settings. This focus not only improves operational efficiency but also enriches the collaborative experience through AI integration.
AI Alignment & Safety: A Comprehensive Approach
Final Thoughts: Cultivating a Holistic View of User Engagement
To truly understand and enhance user engagement in AI products, one must go beyond surface-level metrics. By integrating traditional web metrics with in-depth product experience insights, AI developers can create a user-centric product that not only meets but exceeds user expectations, fostering a loyal and engaged user base.